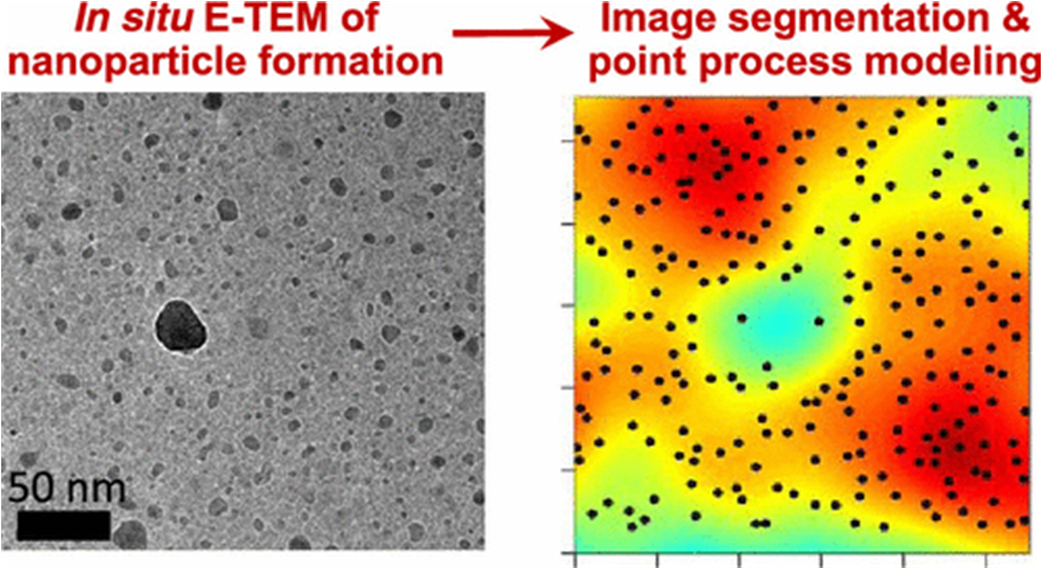
Year
2020Abstract
We combine in situ environmental transmission electron microscopy (E-TEM) with automated image processing and statistical machine learning to uniquely formulate interpretable mathematical models and accurate simulation tools for complex nanoscale phenomena involving coupled physical and chemical processes and interactions that are otherwise hard to model. In particular, there is a need for a better understanding, characterization, and prediction of the proximity effects among dense populations of metal nanocatalysts as they form and evolve over time. Here, we leverage point process theory, a branch of statistical machine learning, to “learn” the spatial dependencies among ensembles of adjacent alumina-supported iron nanoparticles from a time sequence of E-TEM images. We construct a set of point process models to make statistical inferences about the nature of spatial dependencies that govern the rapid formation, or “popping” of nanoparticles during thin film dewetting, concomitant with metal reduction in the presence of acetylene at 750 °C. We show that nanoparticles exhibit strong dispersion behavior, i.e., new nanoparticles pop in dispersed locations at a predictable distance from their existing territorial neighbors. We also show that Gibbs point processes adequately describe the pairwise interactions underlying such time-dependent spatial variations. Further, we build on our machine-learned models to develop a computational simulation tool capable of producing accurate spatiotemporal simulations of nanoparticle formation at finer time resolutions and larger spatial domains than those of experimental observations. This is a much needed capability to overcome current limitations in computational methods supporting the design, analysis, and control of the collective behavior of nanocatalyst populations.